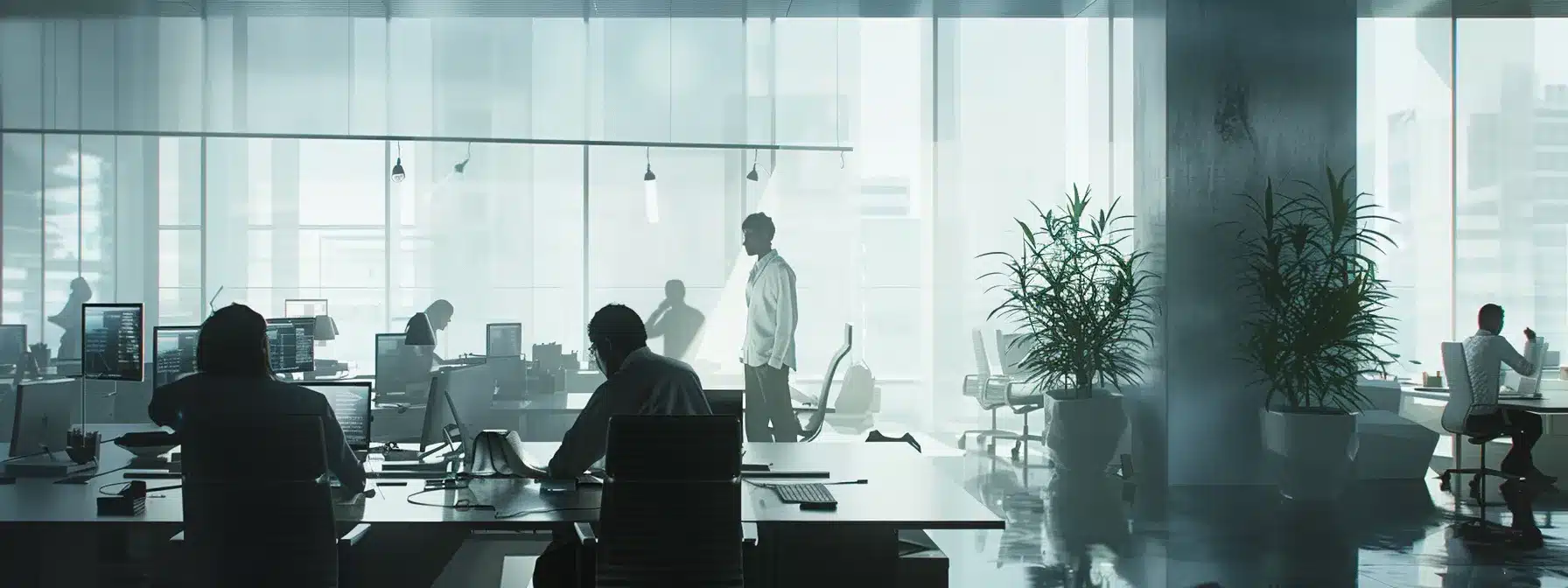
Insurance Coverage Detection Techniques Explained
Insurance coverage detection can significantly improve a healthcare provider’s revenue cycle. Failing to identify all patient insurance can lead to denied claims and increased costs for both providers and patients. This article will cover various types of insurance coverage detection techniques, practical steps for effective implementation, and the challenges professionals may encounter. Readers will learn how these techniques not only minimize claim denials but also streamline administrative processes, ensuring accurate reimbursements. By addressing common issues in coverage detection, this content aims to empower providers to enhance their financial performance and reduce patient out-of-pocket expenses.
Introduction to Insurance Coverage Detection Techniques
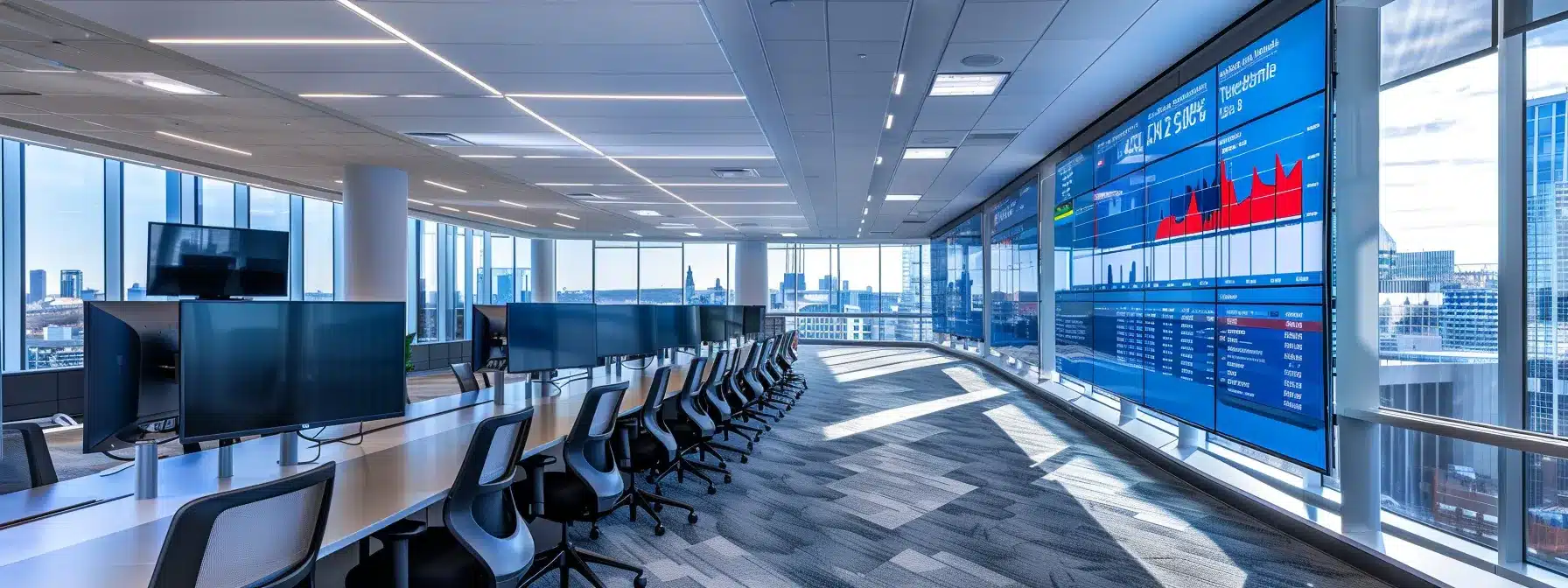
Insurance coverage detection involves assessing and identifying available insurance policies that may benefit health care providers. These techniques are vital in mitigating fraud, including credit card fraud, while enhancing overall revenue outcomes. Key terminology related to evaluation and unsupervised learning will be defined, providing clarity on their relevance to effective coverage detection methods.
Defining Insurance Coverage Detection
Insurance coverage detection refers to the process of identifying and analyzing existing insurance policies that can support healthcare providers in securing accurate reimbursement. By employing advanced techniques from computer science, such as data analysis and machine learning, providers can better manage missing data and uncover potential opportunities for payment. The Office of Inspector General actively monitors these processes to combat medicare fraud, ensuring that effective fraud detection measures are in place and that healthcare practices receive the reimbursements they rightfully deserve.
Importance of Coverage Detection Techniques in Insurance
The importance of coverage detection techniques in insurance cannot be overstated, as they are crucial for ensuring data quality and enhancing claim accuracy. By applying methods such as feature selection, healthcare providers can systematically evaluate existing policies to identify potential gaps or undocumented coverage, improving their financial outcomes. This proactive approach not only streamlines the reimbursement process but also aids in making more reliable predictions about future claims, ultimately supporting providers in securing the payments they deserve while minimizing losses from denied claims.
Key Terminology in Coverage Detection
Key terminology in coverage detection plays a crucial role in effectively identifying potential insurance claims. Terms such as “feature engineering” refer to the process of selecting and transforming variables relevant to consumer data and insurance analytics. Understanding concepts like “univariate analysis,” which focuses on a single variable at a time, allows healthcare providers to pinpoint specific areas of concern, such as drug coverage discrepancies, ensuring comprehensive assessments and improved outcomes.
Types of Insurance Coverage Detection Techniques
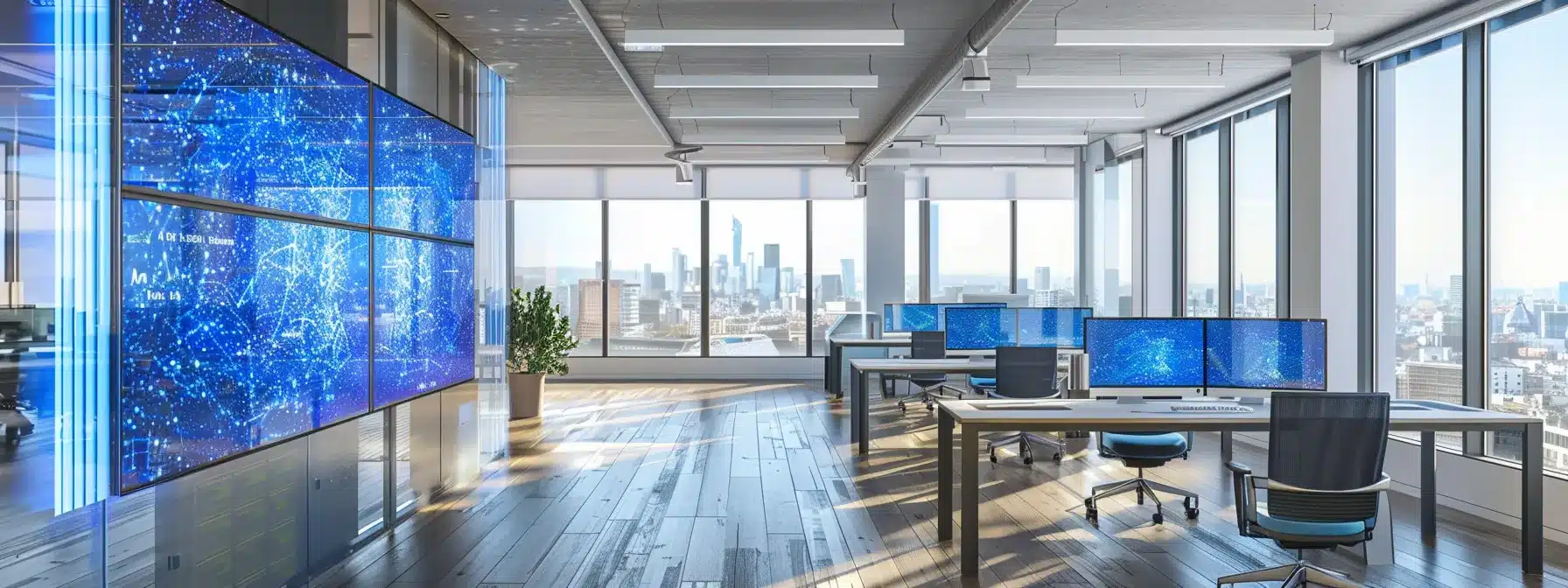
Insurance coverage detection techniques encompass various approaches, including manual and automated detection methods. Data analytics plays a crucial role in examining insurance policies, while machine learning applications leverage statistics, such as receiver operating characteristic metrics, to enhance accuracy. This section will explore these techniques, focusing on their significance in detecting Medicaid claims and addressing potential gaps, including issues related to accident coverage.
Manual vs. Automated Detection Methods
Manual detection methods rely on healthcare providers meticulously reviewing insurance policies and claims through traditional processes, which can be time-consuming and prone to human error. In contrast, automated detection methods harness big data and techniques such as ensemble learning and logistic regression to efficiently analyze vast amounts of information, improving both accuracy and speed. By optimizing the dimension of data analysis, these automated approaches allow insurance companies to quickly identify discrepancies and coverage gaps, ultimately helping providers secure the reimbursements they are owed while minimizing the risk of denied claims.
Data Analytics in Insurance Coverage Detection
Data analytics plays a critical role in detecting insurance coverage by enabling healthcare providers to closely examine claims and identify potential scams. Utilizing techniques like decision trees, providers can analyze vast amounts of data to uncover patterns that indicate discrepancies or gaps in coverage. This approach not only improves the accuracy of claims processing but also safeguards against fraudulent activities, ensuring that practices can secure the reimbursement they are entitled to while maintaining compliance with industry regulations.
Machine Learning Applications in Coverage Detection
Machine learning applications play a vital role in insurance coverage detection, offering advanced techniques for anomaly detection that help identify potential insurance fraud effectively. By analyzing large databases of claims data, machine learning models can recognize patterns and potential inconsistencies, reducing bias in decision-making processes. As a result, these tools empower healthcare providers to combat fraud and secure rightful reimbursements more efficiently, thus addressing critical issues related to crime in the insurance sector.
How to Implement Effective Coverage Detection
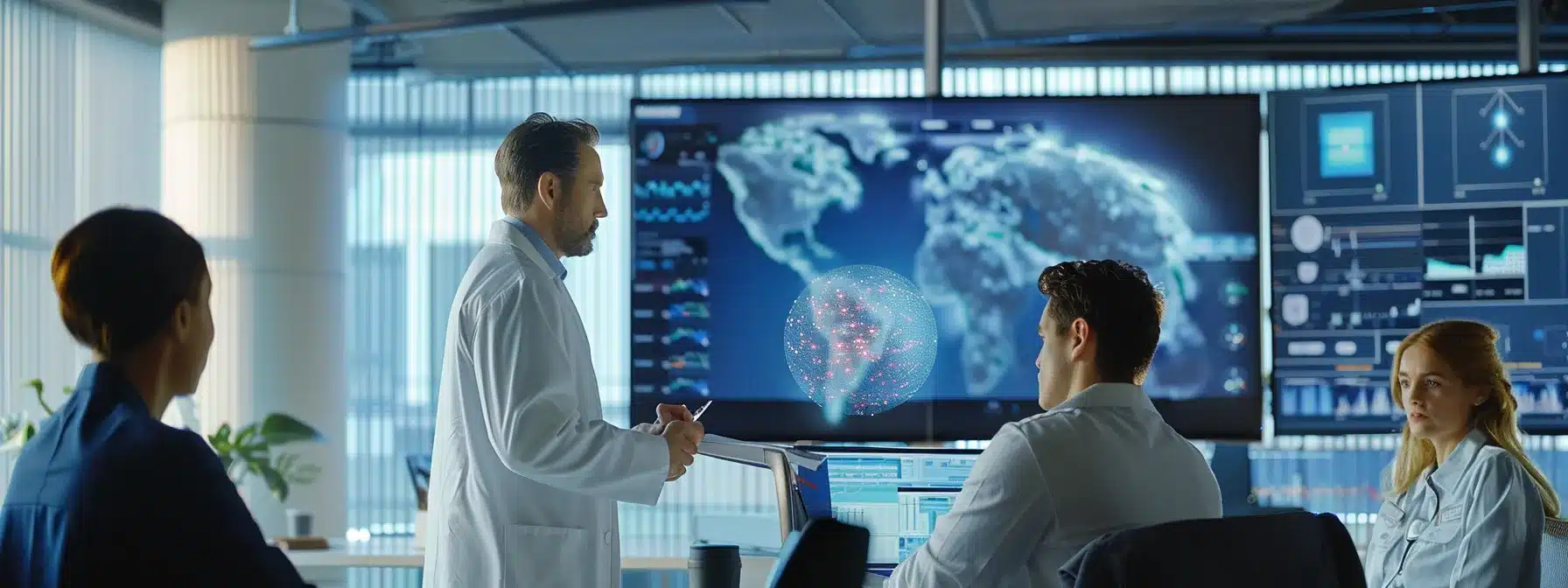
Effective implementation of coverage detection involves several key steps. First, setting up detection techniques must address the complexity and uncertainty inherent in identifying medical prescriptions. Next, integrating technology into the process can reduce false positives and false negatives, enhancing accuracy. Finally, training staff in coverage detection efficiency is essential to ensure they can navigate open access data effectively and maximize outcomes in securing reimbursements.
Steps for Setting Up Detection Techniques
To set up effective coverage detection techniques, healthcare providers must first enable robust data collection frameworks that incorporate informed consent from patients. This ensures that the data utilized in supervised learning models is compliant and ethical, while also addressing privacy concerns. By leveraging advanced intelligence systems, providers can enhance the probability of accurately identifying discrepancies in insurance coverage, thus reducing the risk of claim denials and improving overall financial outcomes.
Integrating Technology Into Coverage Detection
Integrating technology into coverage detection enhances the efficacy of identifying insurance discrepancies and optimizing reimbursement processes. By utilizing machine learning and data science tools, healthcare providers can automate data analysis, facilitating early detection of potential issues that might arise in patient claims. For instance, applications within cancer research have shown how advanced technology can uncover gaps in coverage, allowing providers to address these issues more swiftly and accurately, ensuring that healthcare practices receive the full reimbursement they are entitled to.
Training Staff for Coverage Detection Efficiency
Training staff effectively in coverage detection is essential for improving operational efficiency and minimizing risk in healthcare reimbursement processes. By employing techniques such as sampling and imputation, staff can gain insights into the quality of data used in algorithms, which play a crucial role in identifying discrepancies in patient insurance claims. Practical training that incorporates real-case scenarios, such as those found in clinical trials, enables staff to better understand the implications of these methods, ultimately equipping them to address insurance coverage issues with greater accuracy and confidence.
Challenges in Insurance Coverage Detection Techniques
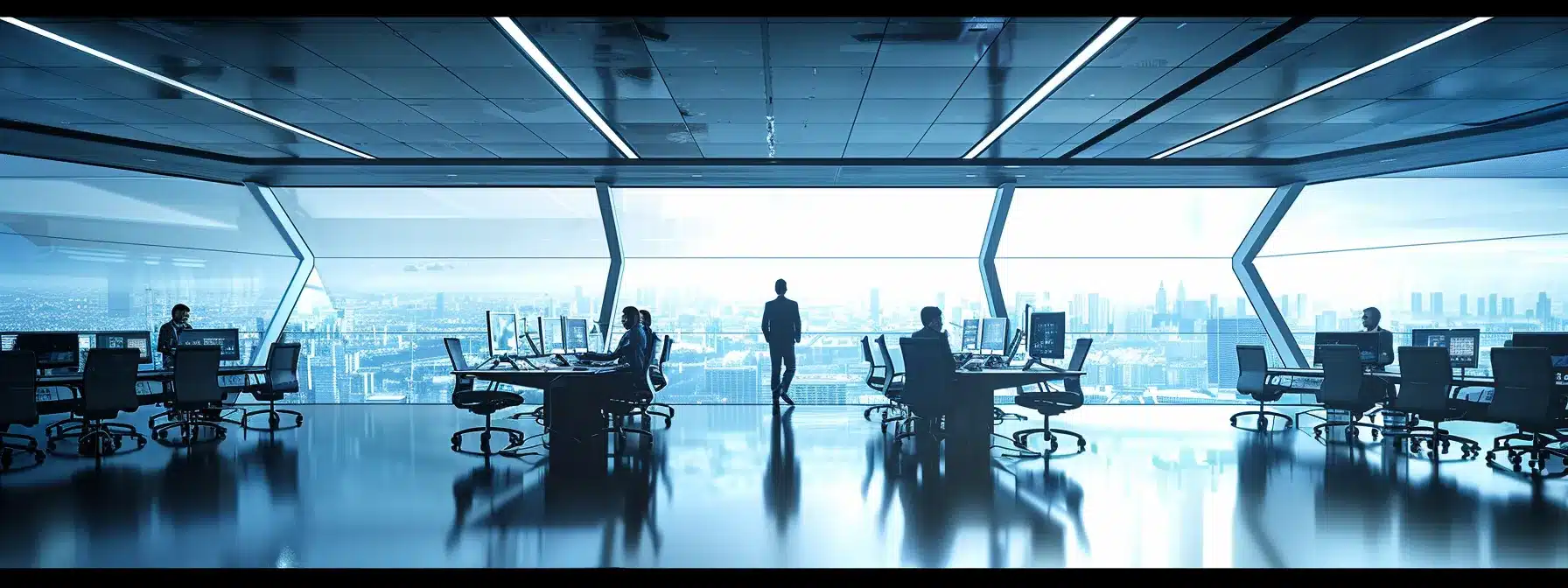
Challenges in insurance coverage detection techniques primarily revolve around common obstacles faced during screening processes, such as computational complexity and data accuracy. Regulatory considerations play a key role in ensuring compliance while maintaining data privacy in detection processes. Addressing these concerns is vital for effectively leveraging anomaly detection techniques, particularly in cases involving injury claims with skewness in reported data, ensuring providers receive the necessary support for accurate reimbursements.
Common Obstacles and Solutions
Common obstacles in insurance coverage detection often stem from the intricate nature of health insurance policies and the variability of patient medication needs. Issues such as incomplete data related to disease conditions or discrepancies in vehicle insurance claims can complicate the reimbursement process. To address these challenges, healthcare providers should adopt comprehensive data management systems that support accurate tracking and analysis of patient claims, ensuring that the information regarding coverage is complete and up to date.
Regulatory Considerations in Coverage Detection
Regulatory considerations are critical in the realm of insurance coverage detection, particularly when addressing potential insurance scams that can impact healthcare providers. Adhering to regulations not only enhances the accuracy of methods like random forest algorithms but also fosters confidence in the outcomes derived from these analyses. By ensuring compliance with industry standards, healthcare providers can mitigate risks associated with fraud while effectively managing therapy-related insurance claims.
Maintaining Data Privacy in Detection Processes
Maintaining data privacy in detection processes is a significant challenge in healthcare, particularly when combating insurance fraud. Regulatory frameworks require strict adherence to guidelines that protect sensitive patient information while conducting research and analysis. To mitigate risks associated with overfitting in machine learning models, healthcare providers must implement robust data governance practices that ensure compliance while effectively monitoring and improving the accuracy of claims processes.
Future of Insurance Coverage Detection Techniques
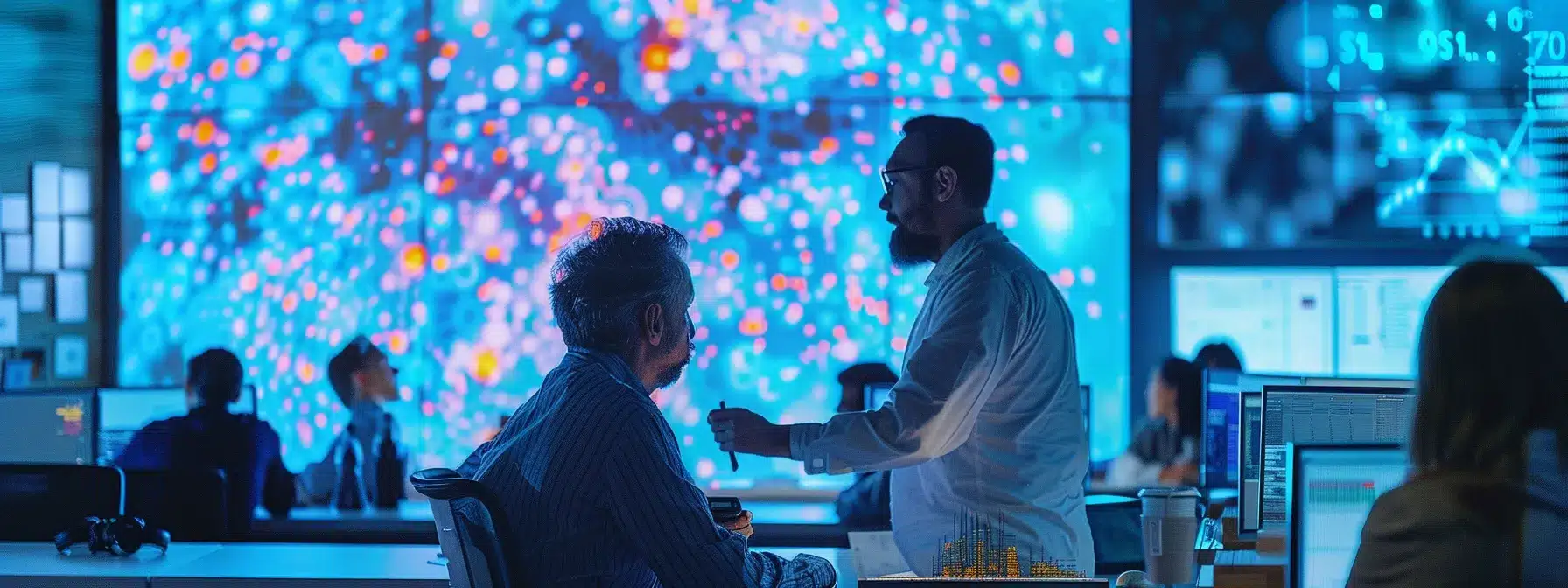
Emerging trends in coverage detection are setting the stage for advancements in the insurance industry. The role of machine learning and data analysis is becoming increasingly significant, as these tools allow physicians to refine parameters for more accurate assessments. With oversight from the Food and Drug Administration, predictions indicate a shift towards more efficient detection techniques, positioning healthcare providers to secure better reimbursements.
Emerging Trends in Coverage Detection
Emerging trends in insurance coverage detection are increasingly focusing on identifying outliers within claims data. By utilizing advanced data analysis techniques, healthcare providers can detect anomalies that may indicate fraud or discrepancies in coverage. This proactive approach enables practices to address potential issues early, ensuring more accurate reimbursements while minimizing the risk of claim denials.
The Role of AI in Shaping Future Techniques
Artificial intelligence (AI) is poised to revolutionize insurance coverage detection techniques by enhancing the ability to analyze vast datasets efficiently. By employing AI algorithms, healthcare providers can identify patterns and anomalies in claims data more accurately, allowing for early detection of potential fraud or gaps in coverage. This technological advancement not only streamlines the reimbursement process but also empowers providers to navigate the complexities of insurance claims with greater confidence, ultimately improving financial outcomes and reducing the risk of claim denials.
Predictions for the Insurance Industry
Predictions for the insurance industry indicate a significant shift towards increased automation and the integration of artificial intelligence in coverage detection techniques. As machine learning becomes more sophisticated, healthcare providers will be better equipped to detect anomalies in claims data promptly, ensuring more accurate reimbursements while minimizing the chances of fraud. This evolution not only enhances operational efficiency for practices but also addresses common pain points related to claim denials, ultimately supporting healthcare providers in navigating the intricacies of insurance processes with greater confidence.
Resources for Further Learning on Coverage Detection Techniques
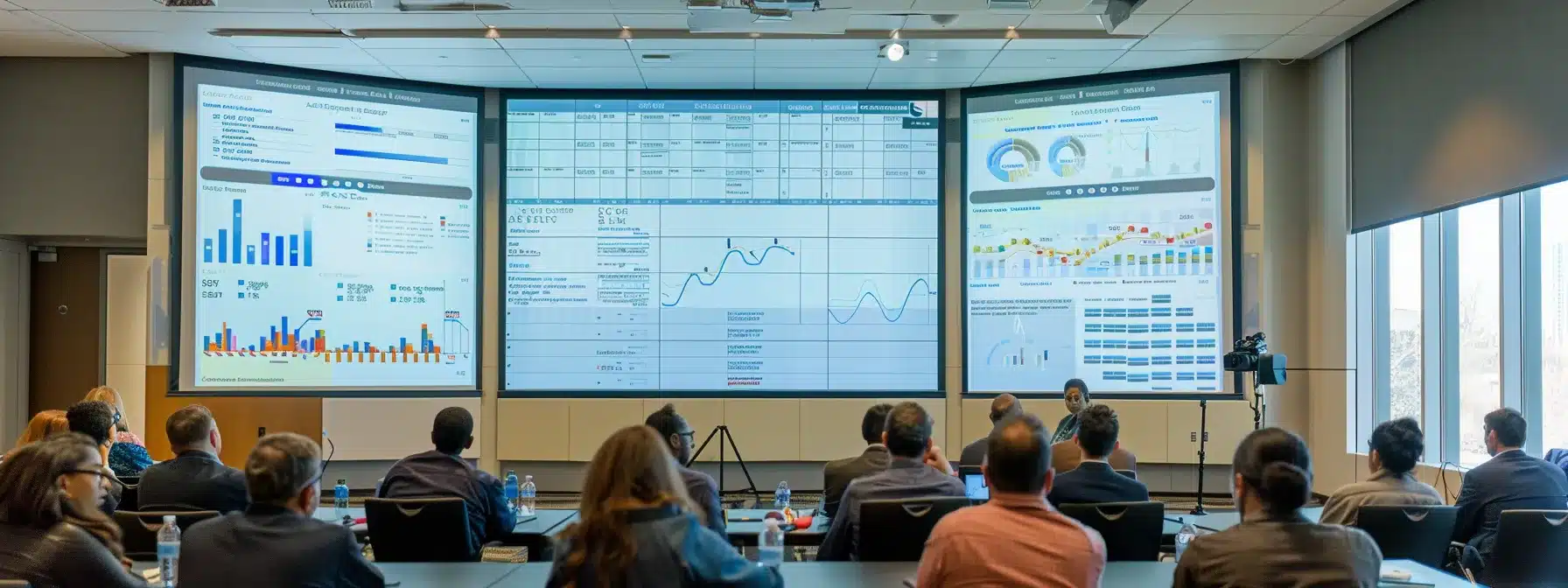
Recommended readings and case studies provide valuable insights into successful insurance coverage detection techniques, highlighting real-world applications and outcomes. Online courses and certifications help healthcare professionals enhance their skills and knowledge in this area. Industry conferences and events present opportunities for networking and learning about the latest trends, ensuring providers stay informed and equipped to tackle the challenges of coverage detection.
Recommended Readings and Case Studies
Recommended readings and case studies on insurance coverage detection techniques offer healthcare providers valuable insights into best practices and real-world applications. Detailed analyses of successful implementations highlight how data analysis and machine learning have enhanced claim accuracy and reduced fraud risk. Engaging with these resources allows practitioners to deepen their understanding of effective strategies, ultimately empowering them to navigate the complexities of insurance processes and improve their reimbursement outcomes.
Online Courses and Certifications
Online courses and certifications provide healthcare professionals with essential knowledge and skills in insurance coverage detection techniques. These educational resources cover crucial areas such as data analysis methods and machine learning applications, empowering providers to enhance their understanding of reimbursement processes. By participating in these programs, individuals can gain practical insights that directly address common challenges within the industry, ultimately supporting their efforts to secure accurate reimbursements and improve financial outcomes.
Industry Conferences and Events
Industry conferences and events provide healthcare providers with valuable opportunities to learn about the latest advancements in insurance coverage detection techniques. These gatherings often feature expert speakers and panel discussions that focus on practical applications of data analytics and machine learning in the healthcare sector. Participating in such events allows professionals to network with peers, share best practices, and gain insights that can directly address challenges they face in securing accurate reimbursements and optimizing operational efficiency.
Conclusion
Insurance coverage detection techniques are essential for healthcare providers to secure accurate reimbursements and optimize financial outcomes. By leveraging advanced data analytics and machine learning, these methods enhance the efficiency of claims processing and reduce the risk of fraud. Implementing effective detection strategies empowers practices to identify discrepancies and maintain compliance with regulations. Ultimately, the proactive approach to coverage detection supports providers in navigating the complexities of insurance processes confidently and effectively.